
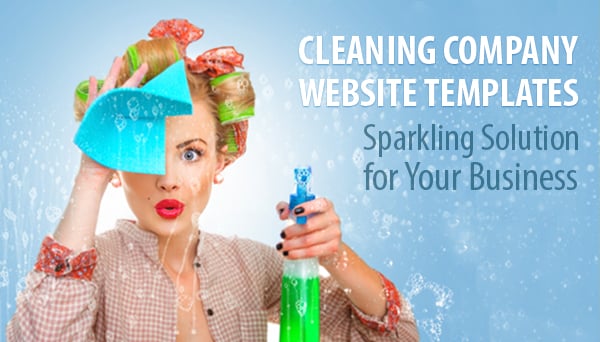
- #SMALL PRIVACY CLEANER PROGRAMS HOW TO#
- #SMALL PRIVACY CLEANER PROGRAMS SOFTWARE#
- #SMALL PRIVACY CLEANER PROGRAMS FREE#
While it is cosmetically similar to spreadsheet software (like Excel), it acts more like a relational database. You can also use it to parse data from online sources. OpenRefine lets you transform data between different formats and ensure that data is cleanly structured.
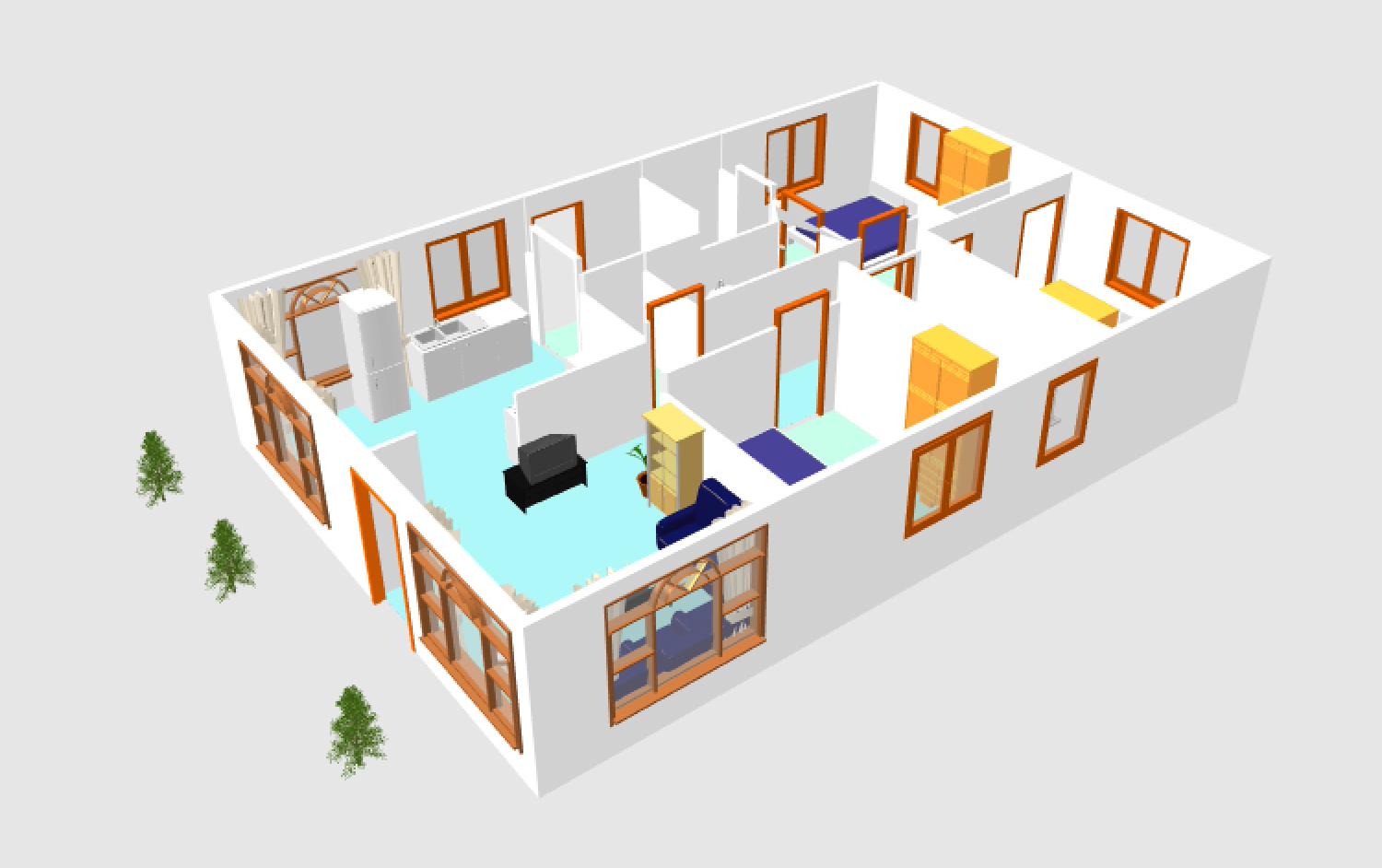
#SMALL PRIVACY CLEANER PROGRAMS FREE#
Its main benefit over other tools on our list is that, being open source, it is free to use and customize. Known previously as Google Refine, OpenRefine is a well-known open-source data tool. Here’s our round-up of the best data cleaning tools on the market right now.
#SMALL PRIVACY CLEANER PROGRAMS HOW TO#
For now, though, check out our top data cleaning tools.Ĭheck out this online workshop we held showing participants how to identify missing values as part of the data cleaning process:įor anyone working with data, the right data cleaning tool is an essential part of your toolkit. For a complete introduction to data cleaning (and why it matters), take a look at this guide.
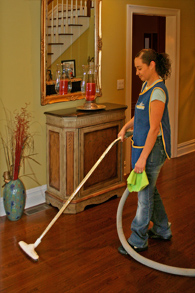
While many of these focus on things like customer data, they can largely be used to clean any kind of big data. Now we’ve recapped what data cleaning involves, let’s take at some of these tools. While tools such as MS Excel, Python, and other scripting languages are all invaluable for data cleaning, there’s an ever-increasing number of vendor data tools available. You’ll often have to go back and repeat some of the earlier steps. It usually involves executing scripts that check if you’ve carried out all the other steps of the process correctly. Validating your data: This is the final step of the process.Dealing with missing data: If there are gaps in your data, what effect will this have? You might choose to remove associated entries, guess missing values, or simply flag them so you can measure their impact later on.For instance, are number fields properly labeled as numerical data? Resolving type conversion and syntax errors: This involves things like removing whitespace, checking for spelling mistakes, or simply ensuring data is categorized correctly.Fixing cross-set data errors: Data rarely comes from a single source ensuring that different data sources don’t contradict each other is vital.You’ll need to make a judgment call about which outliers to keep and which to remove. Removing unwanted outliers: Outliers can be useful, but if they’re erroneous they’ll skew the results of your analysis.Standardizing your data: This involves things like ensuring the numerical observations in your dataset use the same unit of measurement.Unifying the data structure: You’ll need to ensure data from different sources is consistent by mapping it to a unified underlying structure.Getting rid of unwanted observations: Removing observations that aren’t relevant to the problem you’re trying to solve.The main tasks you’ll have to carry out when cleaning data include: What is data cleaning and how is it done? What are some of the most popular data cleaning tools?.Want to skip straight to the tools? Just use the clickable menu. But before we get there, let’s set some context by briefly recapping what data cleaning involves. In this post, we highlight some popular data cleaning tools that data analysts use every day. Better yet, a lot of these have been designed specifically for areas like customer data, which plays a huge part in 21st-century business. Luckily, there are many industry tools available to streamline the process, which can be especially helpful for beginners. If you’re starting as a data analyst, one of the first things you’ll learn is the importance of effective data cleaning.
